Cache Consistency
Overview
In a large number of real-world projects, Redis caches are introduced to alleviate the pressure of database queries. As data is stored in both Redis and the database, there is the issue of data consistency. There is not yet a mature solution to ensure eventual consistency, especially when the following scenario occurs, which directly leads to inconsistencies between the cached data and the database data.
In the above scenario, the final version of the data in the cache is v1, while the final version of the database is v2, which may cause major problems for the application.
Even if you use lock to do the updating, there are still corner cases that can cause inconsistency.
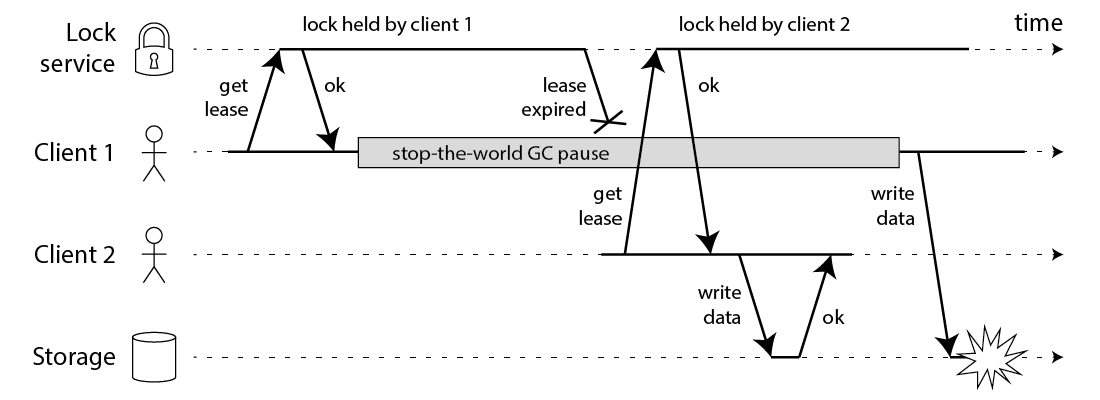
dtm-labs is dedicated to solving the data consistency problem, and after analyzing the existing practices in the industry, a new solution dtm-labs/dtm+dtm-labs/rockscache, which solves the above problem completely. In addition, as a mature solution, the solution is also anti-penetration, anti-breakdown and anti-avalanche, and can also be applied to scenarios where strong data consistency is required.
The existing solutions for managing cache are not covered in this article, but for those who are not familiar with them, you can refer to the following two articles
Problem and Solution
In the timing diagram above, Service 1 has a process suspend (e.g. due to GC), so when it writes v1 to the cache, it overwrites v2 in the cache, resulting in inconsistency (v2 in DB, v1 in cache). What should be the solution to this type of problem? None of the existing solutions have solved the problem completely, but there are several options.
- Set a slightly shorter expire time: within this expire time, it will be inconsistent. The disadvantage is that a shorter expire time means a higher load on the database
- double delete: delete the cache once, delay for a few hundred milliseconds and then delete it again. This approach only further reduces the probability of inconsistency, but it is not forbidden
- Introducing a version like mechanism at the application layer: the application layer has to maintain version, so this solution limit generality and not easily reusable
We have implemented a cache strategy named "tag as deleted" that solves this problem completely, ensuring that data remains consistent between the cache and the database. The solution principle is as follows.
The data in the cache is a hash with the following fields.
- value: the data itself
- lockUtil: data lock expiry time, when a process query the cache no data, then lock the cache for a short time, then query the DB, then update the cache
- owner: data locker uuid
When querying the cache.
- if the data is empty and locked, then sleep for 100ms and query again
- if the data is empty and not locked, execute "fetch data" synchronously and return the result
- if the data is not empty, then return the result immediately and execute "fetch data" asynchronously
The "fetch data" operation is defined as
- determine if the cache needs to be updated, and if one of the following two conditions is met, then the cache needs to be updated
- The data is empty and not locked
- The lock on the data has expired
- If the cache needs to be updated, then lock the cache, query the DB, update and unlock the cache if the lock holder is verified as unchanged.
When the DB data is updated, the cache is guaranteed to be tagged as deleted when the data is successfully updated via dtm (details can be found in a later section)
- TagAsDeleted sets the data expiry time to 10s, and sets the lock to expired, which will trigger a "fetch data" on the next query to the cache
With the above strategy:
If the last version written to the database is Vi, the last version written to the cache is V, and the uuid written to V is uuidv, then there must be a sequence of events as follows.
database write Vi -> cache data tagged as deleted -> some query locks data and writes uuidv -> query database result V -> locker in cache is uuidv, write result V
In this sequence, the read of V occurs after the write of Vi, so V equals Vi, ensuring the eventual consistency of the cached data.
dtm-labs/rockscache already implements the above method and is able to ensure the eventual consistency of the cached data.
- The
Fetch
function implements the previous query cache - The
TagAsDeleted
function implements the "tag as deleted" logic
For those interested, you can refer to dtm-cases/cache which has detailed examples
Atomicity of DB and Cache Operations
For cache management, the industry generally uses a strategy of deleting/updating cached data after writing to the database. Since the save-to-cache and save-to-database operations are not atomic, there must be a time difference, so there will be a window of inconsistency between the two data, which is usually small and has a small impact. However, with the possibility of downtime and various network errors between the two operations, it is possible for one to be completed but not the other, resulting in a long time inconsistency.
To illustrate the above inconsistency scenario, a data user modifies data A to B. After the application modifies the database, it then deletes/updates the cache, and if no exceptions occur, then the data in the database and cache are consistent and there is no problem. However, in a distributed system, process crashes and downtime events may occur, so if a process crashes after updating the database and before deleting/updating the cache, then the data in the database and cache may be inconsistent for a long time.
It is not an easy task to completely resolve the long time inconsistency here, so we present the various solutions below in the following.
Solution 1: Set a Short Expire Time
This solution, the simplest one, is suitable for applications with low concurrency. Developers only need to set the expire time of the cache to a short value, like one minute. This strategy is quite simple to understand and implement, and the semantics provided by the caching system are such that the time window of inconsistency between the cache and the database is short in most cases. When process crash happens, the time window for inconsistency may last for one minute.
For this solution, the database should be able to generate all the accessed cache data in every minute, which may be too expensive for many applications with high concurrency.
Solution 2: Message Queue
This is done by
- When updating the database, simultaneously write a message to the local table. Both operations are in a transaction.
- Write a polling task that continually polls the data in the message table and, send them to the message queue.
- Consuming messages in the message queue and updating/deleting the cache
This approach ensures that the cache will always be updated after a database update. However, this architecture is very heavy, and the development and maintenance costs of these parts are not low: maintenance of the message queue; development and maintenance of efficient polling tasks.
Solution 3: Subscribe Binlog
This solution is very similar to Scenario 2, and the principle is similar to that of master-slave synchronization of databases, where master-slave synchronization of databases is done by subscribing to the binlog and applying updates from the master to the slave, while this solution is done by subscribing to the binlog and applying updates from the database to the cache. This is done by
- deploy and configure debezium to subscribe to the database's binlog
- Listen for data updates and synchronise updates/deletes to the cache
This solution also ensures that the cache will be updated after the database is updated, but like the previous message queue solution, this architecture is also very heavy. On the one hand, debezium is expensive to learn and maintain, and on the other hand, developers may only need a small amount of data to update the cache, which is a waste of resources by subscribing to all binlogs to do this.
Solution 4: DTM 2-phase Messaging
The 2-phase message pattern in dtm is perfect for updating/deleting the cache after modifying the database here, with the following main code.
msg := dtmcli.NewMsg(DtmServer, gid).
Add(busi.Busi+"/DeleteRedis", &Req{Key: key1})
err := msg.DoAndSubmitDB(busi.Busi+"/QueryPrepared", db, func(tx *sql.Tx) error {
// update db data with key1
})
In this code, DoAndSubmitDB will perform a local database operation to modify the database data, and when the modification is complete, it will commit a 2-phase message transaction that will call DeleteRedis asynchronously. QueryPrepared, which ensures that DeleteRedis is executed at least once if the local transaction is committed successfully.
The checkback logic is very simple, just copy code like the following.
app.GET(BusiAPI+"/QueryPrepared", dtmutil.WrapHandler(func(c *gin.Context) interface{} {
return MustBarrierFromGin(c).QueryPrepared(dbGet())
}))
Advantages of this solution.
- The solution is simple to use and the code is short and easy to read
- dtm itself is a stateless common application, relying on the storage engine redis/mysql which is a common infrastructure and does not require additional maintenance of message queues or canal
- The associated operations are modular and easy to maintain, no need to write consumer logic elsewhere like message queues or debezium
Slave cache latency
In the above scenario, it is assumed that after a cache is deleted, the service will always be able to look up the latest data when it makes a data query. But in a real production environment, there may be a master-slave architecture where master-slave latency is not a controllable variable, so how do you handle this?
One is to distinguish between cached data with high and low consistency, and when querying the data, the data with high consistency must be read from the master, while the data with low consistency must be read from the slave. For applications that use rockscache, highly concurrent requests are intercepted at the Redis layer, and at most one request for a piece of data will reach the database, so the load on the database has been significantly reduced and a master read is a practical solution.
The other option is that master-slave separation requires a single chain architecture without forking, so the slave at the end of the chain must be the one with the longest latency. At this point, a binlog-listening solution is used, which requires listening to the slave binlog at the end of the chain, and when a data change notification is received, the cache is tag as deleted in accordance with the above scheme.
These two options have their own advantages and disadvantages, and the business can adopt them according to its own characteristics.
Anti-breakdown
rockscache is also anti-breakdown. When data changes, the popular approaches have the option of either updating the cache or deleting it, each with its own advantages and disadvantages. "tag as deleted" combines the advantages of both approaches and overcomes the disadvantages of both.
Update Cache
By adopting an update caching strategy, then a cache is generated for all DB data updates, without differentiating between hot and cold data, then there are the following problems.
- In memory, even if a piece of data is not read, it is kept in the cache, wasting expensive memory resources.
- Computationally, even if a piece of data is not read, it may be computed multiple times due to multiple updates, wasting expensive computational resources.
- The above-mentioned inconsistency problem can occur with a higher probability.
Delete Cache
Because the previous approach to update caching is more problematic, most practices use a delete cache strategy and generate the cache on demand at query time. This approach solves the problem in the update cache, but introduces a new problem.
- If a hot spot is deleted in a highly concurrent situation, a large number of requests will fail to hit the cache,.
A common approach to preventing cache misses is to use distributed Redis locks to ensure that only one request is made to the database, and that other requests are shared once the cache has been generated. This solution can be suitable for many scenarios, but some scenarios are not.
- For example, if there is an important hotspot data, the computation cost is high and it takes 3s to get the result, then the above solution will delete a hotspot data, there will be a large number of requests waiting 3s to return the result. On the one hand, it may cause a large number of requests timeout, on the other hand many connections are hold in these 3s, will lead to a sudden increase in the number of concurrent connections, may cause system instability.
- In addition, when using Redis locks, the part of the user base that does not get the lock will usually be polled at regular intervals, and this sleep time is not easy to set. If you set a relatively large sleep time of 1s, it is too slow to return cached data for which the result is calculated in 10ms; if you set a sleep time that is too short, then it is very CPU and Redis performance intensive.
Tag as Deleted Method
The "Tag as Deleted" method implemented by dtm-labs/rockscache described earlier is also a delete method, but it completely solves the problem of cache miss in the delete cache, and the collateral problems.
- The cache breakdown problem: in the tag-as-deleted method, if the data in the cache does not exist, then this data in the cache is locked, thus avoiding multiple requests hitting the back-end database.
- The above problems of large numbers of requests taking 3s to return data, and timed polling, also do not exist in delayed deletion, as when hot data is tagged as deleted, the old version of the data is still in the cache and will be returned immediately, without waiting.
Let's see how the tag-as-deleted method performs for different data access frequencies.
- Hotspot data, 1K qps, calculation cost 5ms: the tag-as-deleted method will return expired data in about 5~8ms, while updating the DB first and then the cache will also return expired data in about 0~3ms because it takes time to update the cache, so there is not much difference between the two.
- Hot data, 1K qps, calculation cost 3s: at this time tag-as-deleted method, in about 3s of time, will return expired data. It is usually better behavior to return old data than to wait 3s for the data to be returned.
- Normal data, 50 qps, calculation cost 1s: when the behavior of the tag-as-deleted method is analyzed, the result is similar to 2, no problems.
- Low-frequency data, accessed once every 5 seconds, calculation cost 3s: when the behavior of the tag-as-deleted method is essentially the same as the delete-cache policy, no problems
- Cold data, accessed once every 10 minutes: The tag-as-deleted method and the delete cache policy is basically the same, except that the data is kept for 10s longer than the delete cache method, which does not take up much space, no problem
There is an extreme case where there is no data in the cache and suddenly a large number of requests arrive, a scenario which is not friendly to the update cache method, the delete cache method, or the tag-as-deleted method. This is a scenario that developers need to avoid, and needs to be resolved by warming up the cache, rather than throwing it to the caching system directly. Of course, the tag-as-deleted method does not perform any less well than any other solution as it already minimizes the amount of requests hitting the database.
Anti-penetration and Anti-avalanche
dtm-labs/rockscache also implements anti-penetration and anti-avalanche.
Cache penetration is when data that is not available in either the cache or the database is requested in large numbers. Since the data does not exist, the cache does not exist either and all requests are directed to the database. rockscache can set EmptyExpire
to set the cache time for empty results, if set to 0, then no empty data is cached and anti-penetration is turned off.
A cache avalanche is when there is a large amount of data in the cache, all of which expires at the same point of time, or within a short period of time, and when requests come in with no data in the cache, they will all request the database, which will cause a sudden increase in pressure on the database, which will go down if it can't cope. rockscache can set RandomExpireAdjustment
to add a random value to the expiry time to avoid simultaneous expiration.
Strong Consistency?
The various scenarios for cache consistency have been described above, along with the associated solutions, but is it possible to guarantee the use of cache and still provide strongly consistent data reads and writes? Strongly consistent read and write requirements are less common than the previous scenarios of eventual consistency requirements, but there are quite a few scenarios in the financial sector.
When we discuss strong consistency here, we need to start by making the meaning of consistency clear.
A developer's most intuitive understanding of strong consistency is likely to be that the database and cache are identical, and that the latest writes are available during and after the write, whether read directly from the database or directly from the cache. This "strong consistency" between two separate systems is, to be very clear, theoretically impossible, because updating the database and updating the cache are on different machines and cannot be done at the same time; there will be a time window in any case, during which there must be inconsistencies.
Strong consistency at the application level, however, is possible. Consider briefly the familiar scenarios: CPU cache as the cache of memory, memory as the cache of disk. These are caching scenarios where no consistency problems ever occur. Why? It's really quite simple: All data users are required to read and write data from the cache only.
For DB and Redis, if all data reads can only be provided by the cache, it is easy to achieve strong consistency and no inconsistency will occur. Let's break down the design the cache system for DB and Redis based on their characteristics.
Update Cache or DB First
In analogy to CPU cache or disk cache, both systems modify the cache first and then the underlying storage, so when it comes to the current DB caching scenario should we also modify the cache first and then the DB?
In the vast majority of application scenarios, developers will consider Redis as a cache, and when Redis fails, then the application needs to support degradation processing and still be able to access the database and provide some service capability. In such a scenario, if a downgrade occurs, writing to the cache before writing to the DB would be problematic, as data in Redis may be lost if the data has not been written to DB. Therefore, in a Redis-as-cache scenario, the vast majority of systems would be designed to write to the DB first and then to the cache
Write to DB Succeeded but Cache Failed
What if the process crashes and the write to the DB succeeds, but tag-as-deleted fails the first time? Although it will retry successfully after a few seconds, the user will still have the old version of the data when they go to read the cache in those few seconds. For example, if the user initiates a money transfer and the balances are updated successfully in the DB, and only the update of the cache fails, then the balance seen from the cache is still the old value. The handling of this situation is quite simple: when the writes to the DB is successful, the application does not return success to the user, but waits until the cache updates is also successful and then return success to the user. If the user queries the transfer transaction, they have to query whether both the DB and the cache have succeeded (they can query whether the 2-phase message global transaction has succeeded), and only return success if both have succeeded.
Under the above strategy, when the user initiates a transfer, until the cache is updated, the user sees that the transaction is still being processed and the result is unknown, which match the strong consistency requirement; when the user sees that the transaction has been processed successfully, i.e. the cache has been updated successfully, then all the data from the cache is the updated data, which also match the strong consistency requirement.
dtm-labs/rockscache has already implemented the strong consistency requirement. When the StrongConsistency
option is turned on, then the Fetch
function in rockscache provides a strongly consistent cache read. The principle is not much different from the origin tag-as-deleted method, with the minor change that instead of returning the old version of the data, it waits for the latest result of the `fetch' synchronously
Of course there is a performance penalty for this change, as compared to the eventually consistent data read, a strong consistent read on the one hand has to wait for the latest result of the current "fetch", increasing the read latency, and on the other hand has to wait for the results of other processes, resulting in a sleep wait and consuming resources.
Strong Consistency in Cache Downgrade
The above strongly consistent solution states that the premise of strong consistency is that "all data reads can only be done by the cache". However, if Redis fails and needs to be downgraded, the process of downgrading may be short and only take a few seconds, but this premise is not met, because within those few seconds there will be a mix of read cache and read DB. However, because Redis fails infrequently and applications requiring strong consistency are usually equipped with proprietary Redis, the probability of encountering a failure degradation is low and many applications do not make harsh requirements in this case.
However dtm-labs, a leader in the field of data consistency, has also delved into this problem and offers a solution for such demanding conditions.
The Process of Upgrading and Downgrading
Now let's consider the process of applying a upgrading and downgrading to a problem with the Redis cache. Typically this downgrade switch is in the configuration centre, and when the configuration is modified, the individual application processes are notified of the downgrade configuration change one after another and then downgrade in behavior. In the process of downgrading, there will be a mix of cache and DB accesses, and there may be inconsistencies in our solution above. So how do we handle this to ensure that the application still gets a strong consistent result despite this mixed access?
In the case of mixed access, we can adopt the following strategy to ensure data consistency in mixed access between DB and cache.
- When updating data, use distributed transactions to ensure that the following operations are atomic
- Mark the cache as "locked"
- Update the DB
- Remove the cache "locked" flag and mark it as deleted
- When reading cached data, for data marked as "locked", sleep and wait before reading again; for data marked as deleted, do not return the old data and wait for the new data to complete before returning.
- When reading DB data, it is read directly, without any additional operations
This strategy is not very different from the previous strong consistent solution that does not consider degradation scenarios, the read data part is completely unchanged, all that needs to change is the update data. rockscache assumes that updating the DB is an operation that may fail in business, so a SAGA transaction is used to ensure atomic operations, see example dtm-cases/cache github.com/dtm-labs/dtm-cases/tree/main/cache)
There is a sequential requirement to turn on and off the upgrading and downgrading. It is not possible to turn on cache reads and writes at the same time. So the key point is that, when we reading from cache, we should ensure that all write must both write DB and cache, make the cache providing the newest data.
The detailed process for downgrading is as follows.
- Initial state.
- Read: Mixed reads
- Write: DB+Cache
- Read degradation.
- Read: cached read off. Mixed reads => DB only
- Write: DB + cache
- write degradation.
- Read: DB.
- Write: cache write off. DB+cache => DB only
The upgrade process is reversed as follows.
- initial state.
- Read: DB
- Write: DB
- Write upgrade.
- Read: DB
- Write: write cache on. DB => DB + cache.
- Read upgrade.
- Read: read cache on. DB => Mixed read
- Write: DB + cache
The dtm-labs/rockscache has implemented the above strongly consistent cache management method.
For those interested, see dtm-cases/cache for a detailed example
Summary
This article is long and many of the analyses are rather obscure, so I will conclude with a summary of how Redis caches are used.
- The simplest way: Only set a short cache time, allowing a small number of database changes not synced to the cache.
- Ensure eventual consistency, and to prevent cache breakdown: 2-phase messages + tag-as-deleted(rockscache)
- Strong consistency: 2-phase message + tag-as-deleted (with StrongConsistency enabled)
- The most stringent consistency requirement: 2-phase message + rockscache + downgrading strategy
For the latter three approaches, we recommend using dtm-labs/rockscache as your caching solution